Deep dive into the state of AI & ML. Landscape, trends, regulation. Part 2. Investments, Fundraising, Insights and trends
The AI market is set to skyrocket, reaching a whopping $190.61 billion by 2025. And get this – there will be a staggering 8.4 billion AI-powered digital assistants in existence by 2024, surpassing the global population. With AI advancing at such a breathtaking pace, it’s fascinating and challenging to keep up.
One of the most intriguing trends is the growing utilization of AI tools by investors to enhance their decision-making process and startups to fine-tune their strategies and pitches. These intelligent solutions are reshaping the landscape of both investment and entrepreneurship, unlocking new opportunities and possibilities for growth.
Corporate Investment in 2022, A Look Back
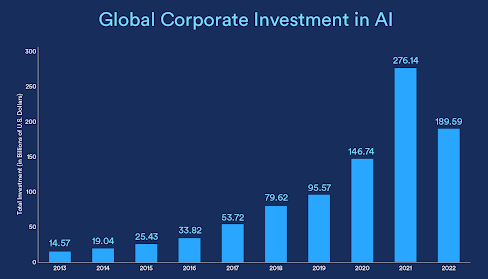
Despite a slight dip from the record highs of 2021, corporate investments, including mergers, acquisitions, minority stakes, private investments, and public offerings, have seen a staggering 13-fold increase over the past decade. Among the notable investment events of the year, the Nuance Communications acquisition stood out as the biggest highlight. Microsoft acquired the computer software tech company for an impressive $19.7 billion, making it a major player in the industry.
In the first quarter of 2023, AI-focused startups raised $17,884,820,266. Among the leaders are OpenAI, SnaboxAQ, Alternyx, Adept AI, and Anthropic.
Exciting Funding Rounds in Generative AI:
- OpenAI received a $10B investment from Microsoft in January 2023
- Runway ML, an AI-powered video editing platform, raised a $50M Series C at a $500M valuation in December 2022
- ImagenAI, an AI-powered photo editing and post-production automation startup, raised $30 million in December 2022
- Descript, and AI-powered media editing app, raised $50M in its Series C in November 2022
- Mem, an AI-powered note-taking app, raised $23.5M in its Series A in November 2022
- Jasper AI, an AI-powered copywriter, raised $125M at a $1.5B valuation in October 2022
- Stability AI, the generative AI company behind Stable Diffusion, raised $101M at $1B valuation in October 2022
- You, an AI-powered search engine, raised $25M in its Series A financings
- Hugging Face, a repository of open source machine learning models, raised $100M in its Series C at a $1B valuation in May 2022
- Inflection AI, AGI startup, raised $225M in its first round of equity financing in May 2022
- Anthropic, an AI research firm, raised $580M in its Series B (investors including from SBF and Caroline Ellison!) in April 2022
- Cohere, an NLP platform, raised $125M in its Series B in February 2022.
- Palo Alto-based SandboxAQ raised $500 million in a venture round. Among the investors are Time Ventures, Breyer Capital, T. Rowe Price, and Eric Schmidt. The enterprise SaaS company provides artificial intelligence and quantum computing solutions
- Alteryx raised $450 million in a post-IPO debt round. The company provides an end-to-end data science and analytics platform for the enterprise
- Adept AI received $350 million in a Series B round led by Spark Capital and General Catalyst. Among the other participants are Microsoft, Nvidia, Greylock, and Frontiers Capital. The startup, founded by former OpenAI engineers, focuses on machine learning research and product lab-building general intelligence.
Several AI-powered metaverse- and web3-focused startups received approximately $87,855,029.
- Among the leaders is Plai Labs, which raised $32 million in a seed round led by a16z. The startup, founded by the former executives of Myspace and Jam City, is developing the next generation of social platforms.
- Gemba raised $18 million in a Series A round led by Parkway Venture Capital. The startup provides a platform for VR workforce training.
- UGC gaming metaverse platform Createra received $10 million in a Series A round led by noted VC firm Andreessen Horowitz. The company enables creators to build engaging 3D worlds and games.
- South Korean startup Com2Verse raised approximately $9,092,400 in a venture round. Hana Ventures, Kyowon Group, and Kyobo Book Center backed the round. On a side note, the metaverse game publisher is a subsidiary of Com2uS.
The Global Artificial Intelligence (AI) market is poised for significant growth between 2023 and 2030, with a positive outlook for 2023 and beyond. As key players in the industry adopt effective strategies, the market is expected to expand further, presenting numerous opportunities for advancement.
Every industry vertical in this fast-paced digital world, benefits immensely from AI & ML tech. Here, we look at the top five sectors:
Healthcare industry
The healthcare sector is poised to experience significant benefits from the advancements in AI in the coming years. With the help of predictive analytics, machine learning algorithms, and computer vision, diseases can be diagnosed more accurately, and personalized treatment plans can be developed to enhance patient outcomes. AI-powered chatbots and virtual assistants will enhance patient engagement and streamline administrative processes, leading to improved overall efficiency.
Also, AI & ML has found a rapidly growing application largely attributed to wearable devices and sensors like fitness trackers and smart health watches. These devices continuously monitor users’ health data, enabling real-time health assessments.
Medical practitioners are benefiting from this technology as well, as it helps them analyze trends and identify potential events that can lead to improved patient diagnoses and treatment. ML algorithms have even shown impressive accuracy in predicting the lifespan of patients with fatal diseases.
AI & ML learning’s contributions extend to two critical areas:
Drug discovery: The process of manufacturing or discovering new drugs is both expensive and time-consuming. Machine learning accelerates various steps in this complex process. For example, Pfizer relies on IBM’s Watson to analyze vast amounts of diverse data for drug discovery.
Personalized treatment: Drug manufacturers often struggle to validate the effectiveness of a specific drug on a large population due to varied responses in clinical trials and potential side effects. To address this challenge, companies like Genentech collaborate with GNS Healthcare, utilizing machine learning and simulation AI platforms to innovate biomedical treatments. ML technology analyzes individual genes to identify patients’ response markers, enabling targeted therapies for better outcomes.
The integration of AI in healthcare will result in more precise diagnoses, cost savings, and increased access to high-quality care, ultimately improving the healthcare experience for patients and healthcare providers alike.
Finance sector
In the finance sector, machine learning technology has become a valuable asset for various financial organizations and banks. It plays a crucial role in combatting fraudulent activities and extracting crucial insights from massive volumes of data. These ML-derived insights assist in identifying investment opportunities, enabling investors to make informed trading decisions.
Data mining methods also contribute to cyber-surveillance systems, helping them detect warning signs of fraudulent activities and effectively neutralizing them. As a result, many financial institutions have entered partnerships with technology companies to harness the advantages of machine learning.
For instance:
Citibank collaborates with the fraud detection company Feedzai to combat online and in-person banking frauds effectively. And PayPal utilizes a range of machine-learning tools to distinguish between legitimate and fraudulent transactions, safeguarding both buyers and sellers.
Retail sector
In the retail sector, machine learning plays a significant role as retail websites use it extensively to provide personalized shopping experiences. ML techniques are employed to capture and analyze customer data, enabling retailers to recommend items based on users’ purchase history. Additionally, machine learning is utilized in marketing campaigns, customer insights, merchandise planning, and price optimization.
According to a report by Grand View Research, Inc. in September 2021, the global recommendation engine market is expected to reach a valuation of $17.30 billion by 2028. Some common examples of recommendation systems in day-to-day life include:
- Amazon: When browsing items on Amazon, the product recommendations on the homepage are powered by machine learning algorithms. Amazon utilizes artificial neural networks (ANN) to provide intelligent, personalized recommendations to customers based on their recent purchase history, comments, bookmarks, and other online activities.
- Netflix and YouTube: These platforms heavily rely on recommendation systems to suggest shows and videos to users based on their viewing history, enhancing the overall user experience.
Furthermore, retail sites also incorporate virtual assistants or conversational chatbots, leveraging machine learning, natural language processing (NLP), and natural language understanding (NLU) to automate and enhance customer shopping experiences.
Travel sector
In the travel industry, machine learning plays a vital role in expanding its horizons. Leading ride-hailing services like Uber and Ola, as well as self-driving cars, rely on robust machine learning backends to enhance their operations.
Take Uber’s dynamic pricing as an example. Their machine learning algorithm, known as ‘Geosurge,’ handles the dynamic pricing parameters. By leveraging real-time predictive modeling on traffic patterns, supply, and demand, Geosurge adjusts fares accordingly. So, if you need to book an Uber in a busy area during rush hour, the dynamic pricing model will come into effect, ensuring you get a ride instantly but at a slightly higher fare.
Furthermore, the travel industry employs machine learning to analyze user reviews. Through sentiment analysis, user comments are classified as positive or negative, aiding companies in the travel sector with campaign monitoring, brand monitoring, and compliance monitoring.
Social Media
Machine learning has revolutionized social media, enabling billions of users to engage efficiently on these platforms. From personalized news feeds to user-specific ads, machine learning plays a pivotal role in driving social media networks.
For instance, Facebook’s auto-tagging feature utilizes image recognition powered by machine learning to automatically identify and tag your friends in photos. The platform uses artificial neural networks (ANN) to recognize familiar faces in users’ contact lists, making automated tagging a seamless experience.
Likewise, on LinkedIn, machine learning algorithms work behind the scenes to suggest the right time to apply for your next role, recommend connections, and assess how your skills rank compared to your peers. These intelligent features are made possible by the power of machine learning.
Self-learning
AI will also be of immense help to individuals who are eager to pursue self-learning using the latest technological aids available to them. As mentioned earlier regarding ChatGPT, this AI model can generate ideas and provide answers to both simple and complex questions. However, to achieve optimal performance, it requires precise prompts and clear instructions. Developing the skill to provide such prompts and instructions becomes crucial for honing self-learning abilities. Once mastered, this opens up endless possibilities for acquiring knowledge by training the brain to break down problems into their core essence and think with clarity to find the most effective solutions. With AI as a valuable tool, individuals can unlock their potential for continuous growth and enhanced problem-solving skills.
Cybersecurity sector
Technology is a double-edged sword, particularly in the realm of cybersecurity where dealing with bad actors is a constant challenge. AI-driven cybersecurity systems have proven to be invaluable in identifying and neutralizing cyber threats by analyzing vast amounts of data and detecting anomalies. Their ability to swiftly respond to potential breaches allows for quicker action to minimize any damage caused. However, as both defenders and attackers employ similar AI technology, safeguarding the AI systems themselves becomes a major concern. Ensuring the integrity and security of these AI-driven defense mechanisms is crucial in maintaining the upper hand in the ongoing battle against cyber threats.
Employment sector
AI’s impact on employment sparks fierce debates, lacking a clear consensus. A Pew Research Center survey revealed 47% believe AI surpasses humans in job application assessment, yet a staggering 71% oppose AI in making final hiring decisions. While 62% foresee a significant workforce impact in two decades, only 28% express personal concern. Though AI may replace some jobs, it also creates new opportunities. AI tools like ChatGPT require human oversight for context and accuracy. For instance, if a company opts for ChatGPT over writers, editors are essential to review AI-generated content for coherence and correctness.
Resource management sector
AI has the potential to revolutionize resource management through optimized allocation, waste reduction, and enhanced sustainability. In water management, AI algorithms analyze data from sensors and satellite imagery to predict water scarcity, optimize irrigation schedules, and detect leakages. AI-powered systems can also optimize waste management, recycling, and circular economy practices, fostering reduced resource consumption and more sustainable use of materials.
As AI becomes increasingly integrated into our lives, giving due importance to ethical considerations becomes crucial. Issues such as privacy, bias, fairness, and accountability pose significant challenges that require careful attention. In Part 3, we delve into this critical aspect of AI.
But what the price of innovation? Big models emit big carbon emissions numbers – through large numbers of parameters in the models, power usage effectiveness of data centers, and even grid efficiency. The heaviest carbon emitter by far was GPT-3, but even the relatively more efficient BLOOM took 433 MWh of power to train, which would be enough to power the average American home for 41 years.
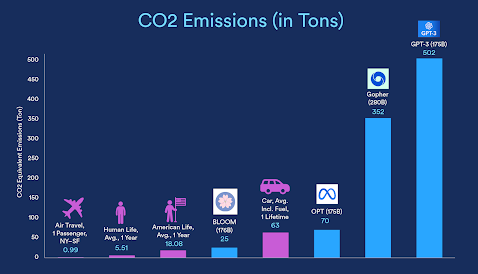
Continue to Part 3. AI Regulation, privacy and other concerns.