Recent Trends and Practices in the Assessment and Rehabilitation of Neurodegenerative Disorders
Introduction
Human gait, the manner in which an individual walks, is a cyclical process comprising various phases. Each step plays a role in essential tasks like weight acceptance, balance maintenance, and limb advancement. Studying human gait in both healthy individuals and those with various pathologies provides insights into movement and balance disorders. Disruptions in the coordinated activity of multiple muscles and the neuro-motor system can lead to atypical gait patterns. Factors such as accidents, aging, and neurological impairments contribute to the degeneration of the musculoskeletal system, resulting in gait abnormalities. These pathological gait issues can significantly impact quality of life by affecting mobility and psychological well-being.
Neurodegenerative disorders, including Parkinson’s disease (PD), multiple sclerosis (MS), Alzheimer’s disease (AD), Huntington’s disease (HD), amyotrophic lateral sclerosis (ALS), and certain forms of dementia, are leading causes of gait impairment. According to a World Health Organization (WHO) prediction in 2006, neurological disorders were anticipated to become the second leading cause of global deaths. However, this milestone was reached much earlier than expected, nearly one and a half decades ahead of the predicted timeframe (Feigin et al., 2017, 2019). PD, a major neurodegenerative disorder of the central nervous system (CNS), affects both motor and non-motor functions, including gait and posture (Emamzadeh and Surguchov, 2018). Additionally, hip and knee issues are significant contributors to non-neurological gait abnormalities (Pirker and Katzenschlager, 2017).
Gait events, such as heel strike (HS) (often referred to as Initial Contact (IC)) and toe off (TO), mark the transition between the stance and swing phases. These events typically appear sequentially in gait timelines, indicating standard gait phases, but they may be absent in certain pathological conditions. Figure 1 illustrates the conventional events and various phases of the human gait cycle. Gait parameters are practical for monitoring and quantifying therapeutic progress (Badaru et al., 2012). Analyzing an individual’s gait can provide insights into kinetic and kinematic aspects and various musculoskeletal functions (Li et al., 2016a). Therefore, gait analysis is crucial in clinical diagnosis, rehabilitation, sports, and biometric security.
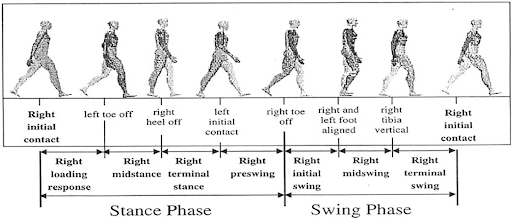
Figure 1. Gait phases and used terminologies to partition the phases. Image source: Li et al. (2016a)
This article reviews the latest advancements in wearable sensors and techniques for ambulatory gait analysis, emphasizing the clinical aspects of neurodegenerative gait disorders. It systematically reports the role of various sensors in studying different facets of clinical gait analysis, including event detection, spatiotemporal parameter measurement, joint kinematics analysis, gait investigation, and postural balance analysis. A thorough discussion of 48 original research articles published from 2005 to 2021, along with several technical and review papers, is provided. Additionally, four commercially available products and their applications are outlined.
Section 2 provides an overview of prevalent neurodegenerative gait disorders and their neuromuscular motor implications. The section “Gait Analysis: Terminologies and Techniques” offers an overview of traditional clinical gait analysis modalities and the shift from stationary ambient sensor-based setups to body-mounted techniques due to advancements in wearable technology. The section “Wearable Sensors for Gait Parameter Estimation” reviews sensing techniques for general and neurodegenerative gait disorders, focusing predominantly on inertial sensors used in various gait analysis applications. Other methods, such as force sensor-based insoles and electromyography, are also discussed in detail. Additionally, the review highlights various miscellaneous sensors that show promising performance. Some commercially available measurement systems are also discussed.
Neurodegenerative Gait Disorders
Neurodegenerative diseases encompass a diverse group of progressive disorders characterized by the degeneration of the central or peripheral nervous system. These diseases disrupt the structural, biochemical, electrical, and functional activities of the nervous system, leading to a loss of neuromuscular coordination and resulting in gait and balance disorders (Cicirelli et al., 2021).
Alzheimer’s Disease
Alzheimer’s disease (AD) is the most prevalent neurodegenerative disorder, primarily impacting memory and comprehension in its early stages and eventually leading to neuromotor impairments. While the exact pathology of AD remains unclear, research has implicated mitochondrial dysfunction (Correia et al., 2012; Carvalho et al., 2015; Rahman and Rhim, 2017) and alterations in axonal transport (AT) (Wang Z. X. et al., 2015) as contributing factors. In advanced stages, common motor impairments include bradykinesia (slow movement), extrapyramidal rigidity, and gait disorders. Patients with AD typically exhibit reduced gait speed and step length, decreased gait frequency (cadence), and increased variability (Pieruccini-Faria et al., 2021). A recent study (Tian et al., 2021) indicates that older adults with slow gait speed and less fragmented activity are at higher risk of developing AD, suggesting that gait could serve as an early indicator for predicting the disease.
Parkinson’s Disease
Parkinson’s disease (PD) is the second most common neurodegenerative disorder, characterized by neuronal cell loss in the midbrain’s substantia nigra pars compacta region and dopamine (DA) depletion in the striatum (ST) (Bastide et al., 2015), along with deficits in multiple neurotransmitters (Marinus et al., 2018). Similar to AD, mitochondrial dysfunction is also frequently observed in PD (Carvalho et al., 2015; Rahman and Rhim, 2017). The accumulation of misfolded α-synuclein, often associated with various neurotoxic pathways, is a distinct hallmark of PD (Chen et al., 2019), contributing to neuroinflammation, oxidative stress, and endoplasmic reticulum (ER) stress. This disorder affects up to 10 million people worldwide (Emamzadeh and Surguchov, 2018). Oxidative stress, resulting from an imbalance in the homeostasis of free radicals, leads to cellular and tissue damage. PD primarily manifests as a hypokinetic movement disorder, causing bradykinesia, hypertonia (rigidity), tremor, and a flexed posture, which are marked by reduced gait speed and step length, and festination (Vallabhajosula et al., 2013). A severe symptom of PD is the freezing of gait (FOG), defined as a brief, episodic absence or marked reduction in the forward progression of the feet while walking, turning, or initiating gait, despite the intention to walk. FOG episodes significantly increase the risk of falls and balance loss in PD patients.
Huntington’s Disease
Huntington’s Disease (HD) stems from a mutation in the Huntingtin (HTT) gene, specifically the expansion of the CAG triplet (cytosine, adenine, and guanine), which results in the elongation of the polyglutamine tract (Xu et al., 2017). Additionally, oxidative stress and the subsequent formation of Reactive Oxygen Species (ROS) are significant contributors to HD (Manoharan et al., 2016). Elevated ROS levels are also associated with the accumulation of proteins such as α-synuclein in Parkinson’s Disease (PD) (Follett et al., 2016). Clinically, HD manifests through involuntary movements like chorea, psychiatric symptoms, and progressive dementia. Patients experience uncontrolled movements and cognitive decline. As the disease advances, uncoordinated body movements and an unsteady gait become more apparent. Characteristics of their gait include slower speed, shorter stride length, variable stepping patterns, and a prolonged stance-to-swing duration ratio. These gait abnormalities significantly increase the risk of falls, further diminishing the patients’ functional abilities (Georgiou et al., 2020).
Amyotrophic Lateral Sclerosis
Amyotrophic lateral sclerosis (ALS) is marked by severe oxidative stress and mitochondrial dysfunction, impacting motor neurons in the cerebral cortex, brain stem, and spinal cord. This disruption in communication between the brain and muscles leads to muscle atrophy and impaired limb function, resulting in altered gait patterns. ALS-related gait dysfunction is characterized by increased variability between strides, reduced single-limb support duration, shorter step length, and decreased walking cadence (Garcia-Gancedo et al., 2019).
Multiple Sclerosis
Multiple sclerosis (MS) is a chronic, progressive, and demyelinating disease characterized by episodic deterioration of neuromuscular functions due to autoimmune-mediated myelin loss and axonal damage (Jones et al., 2017). Clinically, MS presents with sensory, cognitive, and motor impairments. As the disease progresses, patients exhibit distinct gait abnormalities, including reduced gait speed, step length, cadence, and joint range of motion (ROM), alongside increased inter-stride variability. Poor postural control is also common among MS patients (Kalron et al., 2016).
The literature consistently indicates that gait and functional impairments are widespread among individuals with neurodegenerative disorders. Therefore, analyzing gait and balance serves as a valuable clinical tool for diagnosing and managing these impairments. In many instances, such as Parkinson’s Disease (PD), locomotor impairments are among the earliest symptoms. Moreover, different neurological disorders can present with overlapping clinical features, like dementia, making the assessment of movement and balance crucial for accurate diagnosis. Consequently, gait and postural analysis are increasingly utilized in clinical settings for diagnosis, monitoring disease progression, and providing targeted therapy.
Understanding Gait Analysis
Gait analysis is the systematic evaluation of human motion by measuring various parameters such as ground reaction force (GRF), joint torque, range of motion (ROM), and segment acceleration. This process involves a structured approach to collecting and interpreting data, following a strict protocol to ensure consistency. Gait analysis can be broadly categorized into kinematic, kinetic, and spatiotemporal assessments.
Methods of Gait Analysis
In clinical settings, gait analysis can be performed using two main approaches: semi-subjective and objective analysis.
Semi-subjective Analysis:
This approach relies on the expertise of specialists who use predefined observations and questionnaires to assess gait. Patients walk through a designated circuit while clinicians record relevant parameters using basic tools like stopwatches. Although this method is useful in environments lacking advanced equipment, it is often inaccurate, requires significant expertise, and is subjective. It is also difficult to capture multiple parameters simultaneously with this approach.
Objective Analysis:
Objective analysis uses advanced technology to measure multiple gait parameters at once. This includes devices based on image processing, floor-mounted systems, or wearable instruments. These methods provide more accurate and comprehensive data, making them ideal for detailed gait analysis and rehabilitation planning.
Challenges and Innovations in Gait Analysis
Traditional 3D gait analysis, typically conducted with motion capture cameras and force platforms, is effective but expensive and requires specialized knowledge. These factors make it inaccessible for many clinics, especially in rural areas. Additionally, the sheer volume of data produced can be overwhelming for clinicians to interpret. To overcome these barriers, there is a growing demand for affordable, portable, and user-friendly gait analysis tools. Such tools can be widely used in outpatient clinics, community settings, and even at home. Automated, objective gait analysis systems can free up clinicians to focus on developing targeted treatments, ultimately improving patient care. Moreover, these systems can help track patients’ progress and quality of life more effectively.Early investigations into human walking kinematics were pioneered by Marey and Muybridge in the 1870s, utilizing still cameras. Advancements came in the 1970s with the introduction of television cameras, directly linked to computers, expediting the process. This facilitated the development of kinematic gait measurement systems by various research groups, many of which later transitioned into commercial products.
Gait kinetic studies have primarily focused on the forces involved in ground-foot interactions, traditionally measured via force platforms. These platforms have evolved from basic mechanical designs to sophisticated 3D instruments with digital outputs. While early research predominantly centered on ground reaction forces, modern systems now also capture joint moments and powers.
Clinical gait analysis targets gait event detection, spatiotemporal parameter estimation, or gait classification, employing a variety of sensors and systems. These can be categorized into Wearable Sensors (WS) and Non-wearable Sensors (NWS). WS systems are positioned on different body segments, while NWS systems encompass optical motion capture and floor sensor-based approaches.
Optical motion capture systems utilize reflective markers or markerless camera systems to track joint movements and orientations. Marker-based systems determine position and orientation through 3D localization of markers, while markerless systems use human body models and image features. Floor sensor-based methods rely on pressure sensing technology, with force plates measuring ground reaction forces and pressure platforms recording plantar pressure profiles.
NWS systems, including force plates, instrumented walkways, and motion capture systems, are considered gold standards for gait analysis due to their accuracy and repeatability. However, they are costly and require specialized facilities and personnel. Efforts to develop compact and ambulatory WS systems aim to provide alternatives, albeit with reduced accuracy and reliability.
In summary, advancements in gait analysis have progressed from early photographic techniques to sophisticated sensor-based systems, offering insights into human locomotion with varying degrees of accuracy and convenience.
WS offer distinct advantages in providing natural agility during long-term measurements, making them popular for ambulatory gait analysis. This method enables continuous recording of physiological features, meeting the rising demand for technology capable of measuring gait characteristics for activity recognition or gait event classification.
Gait analysis encompasses various dimensions depending on the parameter of interest, including kinematic, kinetic, and physiological aspects, resulting in a diverse set of gait features. Current techniques for extracting these features are broadly classified based on the position of the measurement device, with ambient or non-wearable sensors considered gold standards but facing challenges that limit their clinical translation. Conversely, wearable devices exhibit promising performance for future clinical gait analysis, aiming to address the limitations associated with ambient sensors. This review provides an overview of advancements in wearable technologies geared towards overcoming these challenges.
Wearable gait analysis tools encompass various sensing principles, including inertial sensors (IS), force sensors, flexible goniometers, and myoelectric sensors. Additionally, some studies have explored the use of electromagnetic tracking systems, sensing fabric, ultrasonic sensors, among others. These sensors may be employed individually or in combination (multimodal) depending on the specific application. However, despite the growing interest, wearable gait analysis techniques are still in their early stages, lacking consensus on the essential gait characteristics to assess and their clinical significance.
Inertial sensors, such as Inertial Measurement Units (IMU), consist of accelerometers, gyroscopes, and sometimes magnetometers, capturing an object’s motion dynamics, including orientation, velocity, acceleration, and gravitational forces. Accelerometers measure acceleration along their sensitive axis and are commonly used to capture gait motion. Gyroscopes measure angular rate and can provide information on motion and body segment orientation. The availability of MEMS-based inertial sensors in small form factors, along with advancements in electronic integration, has significantly contributed to the proliferation of research in human movement analysis over the past two decades.
Wearable sensor-based ambulatory gait analysis traces back to Morris’s work in 1973, who utilized six uniaxial accelerometers to solve equations governing the motion of a rotating rigid body and determine its angular acceleration. Since then, IS-based gait analysis has expanded to encompass various measurements, including event detection, kinematic and kinetic parameter estimation, and gait classification. Placing inertial sensors at different limb segments, such as the foot, shank, or hip, yields repeatable signal patterns corresponding to specific gait events. Numerous algorithms and methods, including thresholding, peak detection, zero-crossing, and angular rate reversal, have been developed to identify gait events such as heel strike (HS), toe-off (TO), and flat foot (FF) in real-time or offline modes. For instance, Hundza et al. (2013) employed a single gyroscope placed at the shank to detect TO and HS using rate reversals and zero crossings of the angular rate pattern.
Rampp et al. (2015) introduced a technique for identifying key moments in walking, such as foot strikes and toe-offs, and then computing essential metrics like stride length, stride time, swing duration, and stance time using an accelerometer and gyroscope. They achieved this by integrating the acceleration data from the accelerometer to determine stride length. Joshi et al. (2016) expanded on this work by focusing on toe-off detection during both level walking and walking on inclines using a tri-axial accelerometer positioned on the foot’s dorsum. Their method involved decomposing foot acceleration data through wavelet analysis to pinpoint toe-off events within a specific frequency band. To ensure consistency, they placed foot switches beneath the foot for reference. Their study also addressed the transition from level walking to inclines. However, their algorithm only identified toe-off and lacked validation for heel strikes. Das et al. (2019) proposed a novel approach utilizing an inertial sensor-based wireless foot sensor module (WFSM) to track heel strikes and toe-offs by monitoring foot inclination angles during walking. Their detection algorithm employed peak detection and heuristics to identify these events.
The choice of detection algorithm depends heavily on sensor placement, as signal patterns are closely tied to where sensors are attached on the body. While the methods discussed above are straightforward to implement and demand fewer computational resources and tools, they struggle with detecting gait alterations due to inherent noise in raw data, such as multiple peaks and inertial sensor thresholds. Techniques like Fuzzy Logic, Machine Learning, and Neural Networks have emerged to address these challenges, offering enhanced accuracy and the ability to handle complex signals. However, they often require significant computation time and power, making them less suitable for real-time applications.
While early applications of inertial sensors primarily focused on detecting gait events, recent studies have shifted towards extracting kinematic gait features. The earliest attempts to estimate joint kinematics using accelerometers can be traced back to 1990. Accelerometers have typically been employed for static tilt calculations, while gyroscopes have been used to estimate rotational angles. Combining data from both sensors enables the estimation of joint angles and positions. Figure 3 illustrates the typical functional block diagram for estimating parameters such as linear acceleration, position, and tilt angle using inertial sensors.
Measurement of lower limb joint position and range of motion (ROM) is pivotal in gait kinematics research. These methods typically involve calculating segment kinematics, particularly in the hip and ankle, or determining the relative orientation between proximal and distal sensor-embedded frames for knee joints.
Dejnabadi et al. (2005) introduced an innovative approach to measure the absolute angle of knee rotation by estimating the joint center of rotation acceleration. Placing accelerometers at the shank and thigh regions, they projected their readings to adjacent segments at the center of rotation, thus enabling the direct measurement of knee joint rotation angle. This method eliminates the need for integration or cyclical calculations, reducing integration or drift errors, albeit requiring subject-specific modeling with prior anatomical knowledge.
In recent years, Inertial Measurement Units (IMUs) have found application in studying gait and postural balance, particularly in patients with balance disorders such as Alzheimer’s disease (AD), Parkinson’s disease (PD), mild cognitive impairment (MCI), etc. Hsu et al. (2014) proposed an automated algorithm using a hip-mounted accelerometer to assess gait balance in AD patients and healthy controls. They computed the signal vector magnitude from waist acceleration data and translated step projection displacement into postural sway in both anterior-posterior and medial-lateral directions. This method was validated on AD and healthy control cohorts across various balance test protocols.
Wang Z. X. et al. (2015) developed an automated algorithm utilizing data from three IMUs, placed at the hip and both feet, to conduct the time-up and go test (TUGT). By analyzing motion signature patterns in the coronal plane, they identified stand-up and sit-down events, allowing for the calculation of TUGT performance metrics. Validation of this methodology was conducted on a cohort comprising AD patients and healthy controls.
O’brien et al. (2019) conducted an extensive balance analysis using a single IMU positioned at the hip. They detected gait events, including heel strikes and toe-offs, by analyzing local minima and maxima of continuous wavelet-transformed vertical acceleration. By modeling the lower limbs as inverse pendulums, they estimated spatial features such as step length and extracted frequency-domain features using the Bergs Balance Scale (BBS) for static postural balance assessment, alongside time-domain features derived from acceleration data and its derivatives.
To summarize, Inertial Measurement Units (IMUs), comprising accelerometers, gyroscopes, and magnetometers, offer a promising alternative to traditional gait analysis in laboratory settings due to their numerous advantages. IMU-based methods have been utilized across various aspects of gait measurement, ranging from initial step detection in clinical assessments to automated disease severity quantification in conditions like Parkinson’s disease and balance disorders. The integration of IMU sensor chips into modern smart devices, such as smartphones and wearable bands, signifies a positive trend towards the widespread adoption of these technologies for clinical purposes. However, there are specific challenges that must be addressed to fully realize the potential of IMU-based devices as standard tools for gait analysis. One significant hurdle is the lack of uniformity in technology and its application, leading to a lack of consensus and guidelines for clinical gait evaluation using inertial sensors. Additionally, the preparation of subjects for trials depends on the targeted parameter, posing a challenge for deployment in clinical settings. Moreover, most research focusing on neuro-disorder diagnosis using IMUs tends to be limited to certain disease-specific characteristics, restricting their broader applicability for evaluating other disorders.
Gait Kinematics with Foot Insoles
Traditional floor-mounted pressure measurement systems are precise but costly and limited in recording steps. To address this, researchers developed foot insoles for measuring plantar kinetics wirelessly, allowing longer, more naturalistic gait studies. Some used off-the-shelf sensors, while others custom-built theirs.
Early efforts, like Howell’s, covered the whole foot with sensors, focusing on pressure hotspots. They calibrated each sensor using load cells and analyzed loading patterns during walking. Later, a smaller prototype measured ground reaction forces (vGRF) and joint moments. These insoles, used with motion analysis, accurately modeled gait in stroke patients.
Other systems, like De Rossi’s, used silicon cell-based pads for real-time foot pressure measurement. With 64 elements embedded in an insole, they calculated vGRF and other parameters wirelessly. Their method offers high resolution and temperature insensitivity without extensive calibration.
These pressure insoles are now crucial for studying gait and balance disorders, like freezing of gait in Parkinson’s disease.
Electromyography
Electromyography (EMG) is a method used to measure muscle activity by recording electrical signals produced during contraction and relaxation. These signals, ranging from a few hertz to 400 Hz and voltages from 10 μV to a few millivolts, reflect the underlying chemical processes within muscle cells. EMG can be invasive or non-invasive, with surface EMG (sEMG) being particularly useful for studying dynamic activities like walking and detecting phases of movement.
sEMG has been extensively utilized in various studies, including gait analysis for both healthy individuals and those with neurological impairments. It’s been employed to classify gait phases, detect specific movements like sit-to-stand transitions, and estimate localized muscle fatigue during activities such as walking. Fatigue estimation often involves analyzing the frequency spectrum of EMG signals, with localized muscle fatigue typically causing a downward shift in frequency content.
Research has shown differences in muscle activity between fallers and non-fallers, as well as the ability to detect specific movements like sit-to-stand transitions using EMG data. Additionally, sEMG has been used to evaluate fatigue during tasks like the 30-second sit-to-stand test, particularly in populations with neurological disorders such as hemiplegia, Parkinson’s disease, and multiple sclerosis.
EMG signals have also been correlated with other gait characteristics, such as joint kinematics, providing further insight into movement patterns and muscle function.
Miscellaneous Sensors and Force Myography
Researchers have explored novel sensing techniques for gait analysis, including force myography (FMG). FMG measures external muscle force or pressure generated during movement by using sensors strapped around limbs. Various sensor types such as piezoelectric, capacitive, flexible fabric, and optical sensors have been proposed, with FSRs being the most commonly used.
FMG has been utilized primarily for upper limb movements, with studies showing its effectiveness in classifying different walking types and its correlation with surface muscle electrical activity. Some research suggests that FMG signals may provide better indicators of fatigue compared to sEMG signals.
Recent studies have focused on using FMG for gait event and phase detection. Godiyal et al. (2018) developed a method using FSRs strapped around the thigh to detect heel strike (HS) and toe-off (TO) during overground and ramp walking. Jiang et al. (2018) utilized an FMG band around the ankle to classify gait phases during treadmill walking based on distinctive pressure distribution patterns recorded by the sensors. These studies demonstrate the potential of FMG in gait analysis, although subject-specific databases may be needed for accurate detection.
Piezoelectric Sensors
Piezoelectric sensors create electric potential under stress and are commonly used for dynamic pressure and vibration measurement. They’re typically made from ceramic or MEMS materials. Ceramic sensors aren’t ideal for static or low-frequency measurements due to parasitic effects. MEMS sensors offer advantages like 3-axis measurement capability and generating electrical impulses based on force direction. They’re great for detecting human motion due to their flexibility and wide frequency range.
Balbinot et al. (2014) developed a footswitch system for detecting heel strike (HS) and toe-off (TO) using a piezoelectric transducer from a buzzer. The sensors were calibrated and placed beneath a rubber insole at the hallux and heel. HS and TO were identified when force exceeded or fell below a 5N threshold, used to derive gait parameters, validated with a standard force plate.
Cha et al. (2018) reported gait recognition using flexible piezoelectric sensors (PVDF). Four sensors attached to loose clothing at knee and hip joints generated electric voltage corresponding to joint bending. The system extracted periodic motion components from signals to correlate with gait speed and detect postural transitions.
While many piezoelectric sensor-based gait systems focus on analyzing vertical force, shear stress is crucial, especially for pathological gait. Crosstalk from piezoelectric coefficients affects measurement accuracy. Recent approaches include using multilayer structures with piezoelectric films in distinct orientations, lamination techniques for electrodes, and signal decomposition for accurate measurement.
Wearable microphones
Wearable microphones are gaining traction for capturing footstep sounds during walking, offering insights into gait analysis. Wang et al. (2016) introduced a method using ankle-mounted microphones to detect footsteps, heel strikes (HS), and toe-offs (TO). They extracted 36 features from spectral analysis, employing a support vector machine (SVM) for classification and achieving 95% accuracy in healthy subjects.
Jesus et al. (2019) estimated gait parameters for a Parkinson’s disease subject using a microphone, validated against motion capture. Ferlini et al. (2021) proposed “EarGate,” utilizing an inward-facing earphone to identify gait from bone-propagated sounds in the ear canal with over 97% accuracy. Chiang et al. (2019, 2020) explored using microphones and buzzers on shoes to detect gait events and compute step characteristics.
Ultrasonic sensors
Ultrasonic sensors gauge object distance by emitting high-frequency sound waves and measuring their echo. This time-of-flight method is adept at minimizing background noise compared to intensity-based measurements, making it ideal for precise distance calculations, even in gait analysis.
Huitema et al. (2002) devised a hybrid system with stationary ultrasonic transmitters and receivers on shoes, measuring foot position and velocity to calculate step and stride length. Gait events like heel strikes and toe-offs were determined from speed plot variations, validated on four subjects.
Qi et al. (2014) implemented a wireless ultrasonic network fixed on shoes and room planes to track 3D foot trajectories. Validated against motion camera systems, it achieved a 4.2% RMS error in tracking foot movements.
Multimodal In-Shoe Approaches
Researchers are integrating various techniques to develop wearable gait analysis systems, especially those based in shoes, aiming to capture key kinetic and kinematic parameters efficiently. These shoe-based systems offer quick setup and discreet integration of electronics, making them appealing for everyday use. One pioneering example is GaitShoe by Bamberg et al. (2008) from MIT, which combines accelerometers, gyroscopes, foot-force sensors, bend sensors, pressure sensors, and electric field height sensors. This comprehensive system measures parameters like step length, velocity, foot orientation, force distribution, gait events, and foot clearance. Although effective, it requires significant customization.
A more recent example is eSHOE by Jagos et al. (2017), featuring a wireless system with a six-axis IMU and four force-sensitive resistors (FSRs) for calculating temporal parameters like gait cycle time and cadence. Validated against the GAITRite® walkway system, eSHOE shows promising correlation in measuring parameters related to step timing. However, its focus on temporal features limits its capacity for capturing spatial characteristics crucial for clinical gait analysis. Similar multimodal in-shoe systems have been developed for rehabilitation tasks, highlighting the versatility and potential of this approach.
Commercial Ambulatory Wearable Gait Sensors
While research in wearable sensor-based gait analysis has flourished, commercial systems are still limited. Notable among them is the Xsens Motion System, renowned for capturing full-body kinematics using inertial trackers wirelessly. It’s extensively employed in gait studies and biomechanics research, serving as a benchmark for custom-developed gait measurement methods.
APDM Wearable Technologies offers “Mobility Lab,” a recent product for gait and biomechanics analysis. It conducts standardized tests, generating patient reports for clinical assessment, covering gait parameters, arm kinematics, postural sway, and sit-stand motions.
F-Scan is an in-shoe plantar pressure measurement system providing dynamic pressure and force data, catering to both researchers and clinicians. It aids in therapy optimization and foot biomechanics understanding, with customizable reports for medical practitioners.
Moticon presents an insole-based plantar force measurement system integrated with IMU, offering entirely wireless data collection. Its unobtrusive design allows embedding in any shoe, enabling continuous outdoor data recording. These commercial solutions facilitate diverse applications in gait analysis and biomechanics research.
Advances in Therapeutic Intervention
Current pharmacological treatments for neurodegenerative disorders offer symptomatic relief but lack efficacy in curing or preventing these conditions, often leading to adverse effects. For instance, Levodopa, commonly prescribed for Parkinson’s disease, can cause dyskinesia and symptom relapse. Given the diverse mechanisms underlying neurodegenerative disorders and their similar symptomatic presentations, there’s a pressing need for targeted interventions. Researchers are exploring innovative approaches such as wearables, virtual reality, and phytochemicals to enhance neuro-motor functions and potentially slow disease progression.
Wearable and Virtual Reality Technologies for Gait and Balance Rehabilitation
Wearable devices offer real-time assistance for neurological gait disorders by analyzing kinematic and physiological data and providing immediate feedback cues. They engage patients in continuous rehabilitation, promoting motor learning. Lightweight and portable, wearables are suitable for long-term outdoor use, allowing realistic scenario practice.
Virtual reality (VR) revolutionizes neurological rehabilitation by immersing patients in interactive virtual environments. Unlike traditional physiotherapy, which can be monotonous, VR turns therapy into engaging games. Real-time body movement data act as biofeedback to adapt game environments, ensuring patient involvement and enhancing therapy effectiveness.
Phytochemicals and Its Neuroprotective Roles
Given the limitations of existing drugs for neurological disorders, researchers are turning to plant-derived molecules, or phytochemicals, for potential cures and preventative measures. These compounds target key pathological features such as oxidative stress, neuro-inflammation, and protein aggregation. For instance, phytochemicals like Resveratrol and Melatonin have shown promise in improving mitochondrial function, a primary factor in diseases like Alzheimer’s and Parkinson’s. Apigenin, known for its anti-inflammatory properties, has been found to alleviate α-synuclein accumulation and mitochondrial dysfunction in Parkinson’s disease rats. Studies also indicate the effectiveness of compounds like Berberine, quercetin, ferulic acid, and Curcumin in improving motor functions and cognitive abilities in neuro-disorder induced animal models. For example, blueberry supplementation has been linked to improved mobility and daily activities in the elderly, while Berberine showed efficacy in preventing memory loss in Parkinson’s disease and alleviating motor dysfunction in Parkinson’s and Huntington’s disease models. Quercetin enhances antioxidant enzyme activity, improving motor function in Parkinson’s disease, and Curcumin, known for its antioxidant properties, shows promise against Alzheimer’s and Parkinson’s by inhibiting β-amyloid aggregation and protecting mitochondria. Ginseng has also demonstrated improvements in dopaminergic neuronal loss and gait disturbance in Parkinson’s disease models.
Gait and balance analysis not only help understand locomotor impairments but also aid in accurate diagnosis of neurodegenerative disorders like Parkinson’s, Alzheimer’s, and multiple sclerosis, which often present similar non-motor symptoms. Conventional gait analysis setups, though accurate, are costly, bulky, and require specialized operators. To address these limitations, researchers globally have explored wearable sensor (WS)-based gait analysis since 2005, employing technologies such as IMU, pressure sensors, EMG, FMG, piezoelectric sensors, microphones, and ultrasonic sensors, along with commercial systems. These sensors play crucial roles in assessing various clinically relevant aspects of neurodegenerative gait disorders. Ongoing efforts focus on mitigating these disorders through wearable and virtual reality technologies, alongside exploring the potential of phytochemicals in alleviating symptoms.
Event Detection
Gait events such as heel strike (HS), toe-off (TO), and foot flat (FF) are crucial for segmenting gait and indicating transitions between walking states. Various sensors, including IMUs and EMG, have been explored to detect these events in both healthy and impaired gait. Detection methods commonly used include thresholding (for IMUs, footswitches, pressure sensors, wearable microphones, and piezoelectric sensors) and techniques like rate reversal, peak detection, and zero-crossing (for IMUs). Additionally, PSD and frequency analysis are employed for EMG and FMG. Researchers have also turned to machine learning to handle complex signals from IMUs for accurate event recognition in impaired gait.
Kinematic Analysis
IMUs play a predominant role in studying the biomechanics of walking, offering insights into joint and limb trajectories. They are utilized extensively for tasks ranging from head to toe movement analysis to pose estimation. Additionally, there’s a recent focus on analyzing joint angles using instrumented force insoles, with efforts to employ artificial neural networks for this purpose.
IMUs play a predominant role in studying the biomechanics of walking, offering insights into joint and limb trajectories. They are utilized extensively for tasks ranging from head to toe movement analysis to pose estimation. Additionally, there’s a recent focus on analyzing joint angles using instrumented force insoles, with efforts to employ artificial neural networks for this purpose.
Kinetic Analysis
Understanding forces and torques in walking is crucial, with ground reaction forces being a key kinematic parameter in gait analysis. Researchers commonly investigate force and pressure sensors embedded in footwear for both healthy and impaired gait studies. Recently, there has been interest in using inertial sensors to estimate ground reaction forces and joint moments. Additionally, EMG and FMG are commonly employed techniques for measuring muscle forces during walking.
Spatiotemporal Analysis
Gait analysis involves assessing both temporal and spatial parameters crucial for quantitative and qualitative evaluation. While various sensors are useful for temporal parameter measurement through event detection, IMUs integrated with human models are widely utilized for estimating spatial parameters such as step/stride length and step width.
Balance Analysis
Postural balance, indicated by factors like center of mass (CoG) and postural sway, is crucial for assessing gait and stance. Foot insoles with sensors are commonly used to measure these factors, although some studies also utilize IMUs. These methods can assess balance scales such as 10 MWT, BBS, 2 min standing test, and TUGT. However, wearable devices for such analyses in non-specialized clinical settings are limited. To implement them effectively, reducing the number of sensors is essential for long-term monitoring, as more sensors increase complexity and cost. The output from gait analysis systems should include clinically meaningful features, minimizing the burden on clinicians. Although commercially available systems offer accepted parameters, data interpretation lacks objectivity and relies heavily on clinician expertise. Automating this process to correlate normal and pathological gait could improve evaluation. Recent research has focused on using gait features to classify neurodegenerative disorders, but much of it relies on lab-based systems.
Therapeutic Advances
Neurodegenerative disorders like PD, AD, MS, ALS, etc., share common hallmarks such as mitochondrial dysfunction, α-synuclein accumulation, Amyloid-β plaque formation, dopamine depletion, neuroinflammation, and oxidative stress. Bioactive compounds like Curcumin, AGN, and Resveratrol show promise in mitigating these pathologies through antioxidant, anti-inflammatory, and mitochondrial function improvement properties.
Aside from pharmacological treatments, efforts are being made to address motor challenges in neuro-disorders through VR and wearable devices. These devices analyze body movements in real-time and provide countermeasure signals, facilitating motor and cognitive learning for effective long-term rehabilitation.
Future Direction
Wearable gait analysis has made significant strides in recent years, with products like XSENS F-Scan entering the market. However, for broader clinical applicability, several improvements are needed.
Firstly, standardization is crucial. There’s currently no consensus on measurement methods or outcome measures, hindering widespread adoption. Devices must produce reliable and reproducible results for clinical and research use.
Secondly, intelligent algorithms are needed for diagnosing various disorders. Most existing algorithms are disease-specific and lack efficiency for broader clinical use. Additionally, there’s a need for grading disease severity rather than just binary classification, which would aid in prescribing targeted treatments.
Thirdly, smartphone-based analysis deserves more attention due to its widespread availability. Mobile technology can provide gait analysis to a larger population, potentially revolutionizing clinical practice.
In conclusion, recent advancements have shown promise in assessing and treating neurodegenerative gait disorders. Wearable sensing techniques offer valuable insights, especially IMUs, but challenges remain in standardization and clinical integration. Biofeedback systems and VR technology also hold potential for rehabilitation, while research on phytochemical compounds shows promise but requires further investigation.